A new cyber attack called 'slop squatting' may emerge that exploits hallucinations caused by code-generating AI
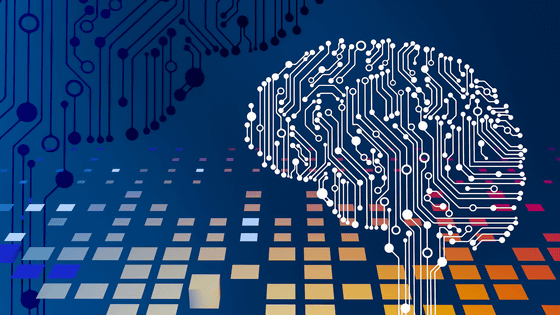
The widespread use of AI-based code generation has greatly improved development efficiency, but it has also created entirely new risks. Large-scale language models (LLMs) have the risk of generating 'non-existent package names' as hallucinations, and it has been pointed out that this could lead to a new type of software supply chain attack called ' slop squatting ,' in which attackers publish malicious packages with the same names to deceive developers who write code with AI assistance.
[2406.10279] We Have a Package for You! A Comprehensive Analysis of Package Hallucinations by Code Generating LLMs
The Rise of Slopsquatting: How AI Hallucinations Are Fueling...
https://socket.dev/blog/slopsquatting-how-ai-hallucinations-are-fueling-a-new-class-of-supply-chain-attacks
AI-hallucinated code dependencies become new supply chain risk
https://www.bleepingcomputer.com/news/security/ai-hallucinated-code-dependencies-become-new-supply-chain-risk/
Unlike traditional typosquatting attacks, which target users' typos, slopsquatting attacks exploit AI errors. For example, tools like ChatGPT and GitHub Copilot may recommend non-existent packages, leading to uncritical copying and pasting and installation, which could lead to malware being unintentionally introduced into a project.
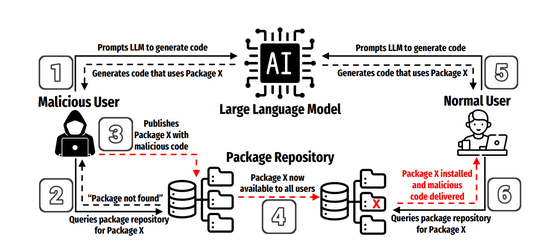
A joint research team from the University of Texas at San Antonio, the University of Oklahoma, and Virginia Tech generated and analyzed a total of 576,000 Python and JavaScript code samples from 16 types of LLMs, including commercial and open source. As a result, it was found that 19.7% of the packages were missing, that is, hallucinations. In particular, the open source model showed a high hallucination rate of 21.7% on average, and some CodeLlama models were reported to have hallucinations in more than one-third of cases. In contrast, the hallucination rate of GPT-4 Turbo was only 3.59%, making it a relatively stable model.
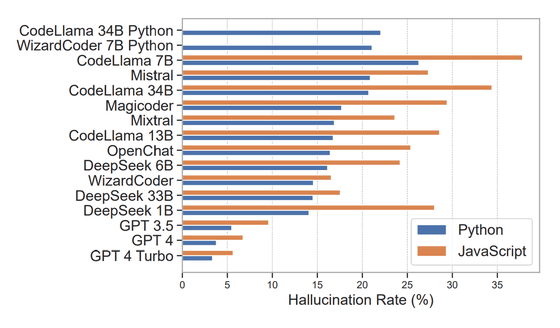
Then, when the 500 hallucination generation prompts were re-run 10 times each, 43% repeatedly generated the same hallucination in every trial, and 39% never re-generated it. The research team argues that this indicates that the hallucinations are not completely random, but rather have some regularity or model-specific quirks. In other words, an attacker can efficiently identify the package name to target by simply observing the AI output a few times.
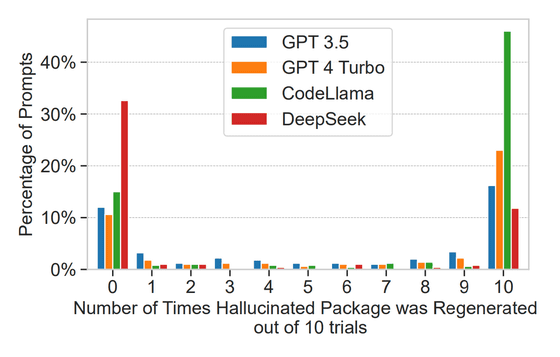
In addition, it was confirmed that the hallucination rate increased dramatically when the temperature variable, which controls the randomness of the generation, was increased. In one model, the research team reported that at high temperature settings, the model output more hallucinatory packages than valid packages. The team also found that models with a large number of proposed packages and greater redundancy tended to have a higher hallucination rate, while models that only used reliable packages tended to have fewer hallucinations.
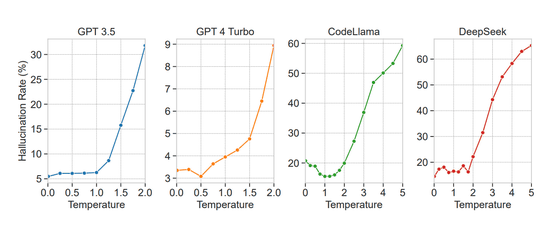
Many of the hallucinated packages looked very similar to the real thing, with 38% showing moderate similarity to existing packages, 13% showing simple typos, and many of the rest having entirely fictitious but plausible names. The team also found cases of confusion between Python and JavaScript, highlighting the risk of misidentifying dependencies between languages.
In addition, it was found that some models, such as GPT-4 Turbo and DeepSeek, have the ability to determine with an accuracy of over 75% whether the packages they generate are hallucinations. This suggests that it is possible to take 'self-verifying' measures that reevaluate the output, and the research team argued that this is a promising clue for suppressing hallucinations in the future.
In recent years, a style of 'vibe coding' has become popular, where users do not write detailed code, but simply tell the AI what they want to do, and the AI generates the code. As the output of AI tends to be accepted uncritically, the risk of hallucinated packages being used in actual development sites has increased dramatically.
Security company Socket pointed out that behind the convenience of code-generating AI lies a serious security risk, and that this threat is certainly becoming a reality. They stated that in the future, it will be necessary to combat new attack methods from both the perspective of developer literacy and countermeasure tools.
Related Posts:
in Security, Posted by log1i_yk