'Meaning Machine' visualizes how large-scale language models break words down into tokens and process them
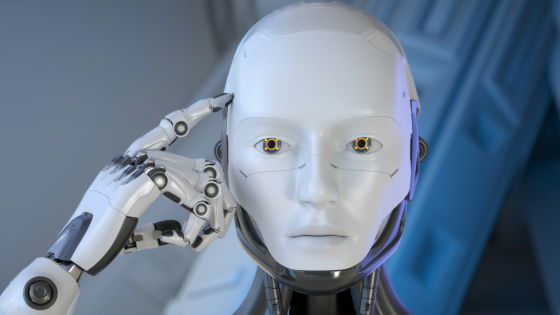
Generative AI using large-scale language models such as ChatGPT, Claude, and Grok respond appropriately to user words just like a human. However, there is a big difference between how large-scale language models process language and how humans process language. The website ' Meaning Machine ' provides a visually easy-to-understand view of how large-scale language models process language.
Meaning Machine · Streamlit
How Language Models See You - by Joshua Hathcock
https://theperformanceage.com/p/how-language-models-see-you
When you access the Meaning Machine, a form for entering a sentence will be displayed at the top of the screen. By default, the sentence 'The young student didn't submit the final report on time' is displayed.
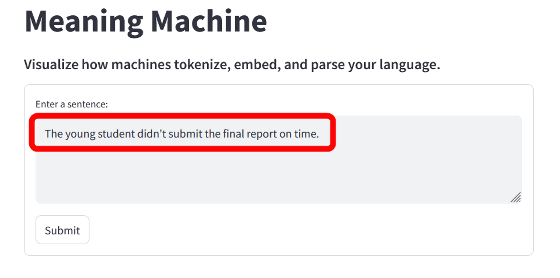
Below that, the input sentence is split into words, and each word is shown with a numeric ID when it is represented as a '
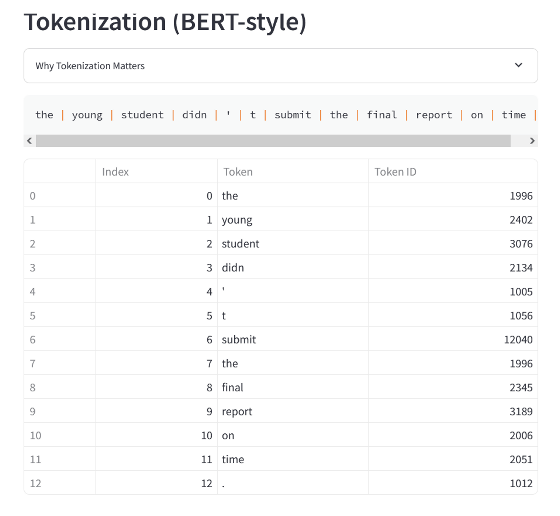
Joshua Hathcock, the developer of Meaning Machine, explains that large-scale language models do not process entire sentences together, but rather split words and character sets into numerical IDs called tokens and process them abstractly. For example, in the case of the GPT model on which ChatGPT is based, common words such as 'The,' 'young,' 'student,' 'didn,' 't,' and 'submit' are often represented by a single token, but rare words are split into multiple tokens made up of combinations of subwords.
The large-scale language model then identifies the grammatical role of each token and infers the subject, verb, object, etc. of the sentence. In the example sentence, the subject is 'student,' the verb is 'submit,' and the object is 'report.'
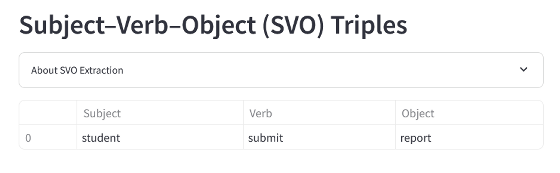
The large-scale language model tags each token with its part of speech (POS), maps dependencies in a sentence, and structures and represents the sentence.
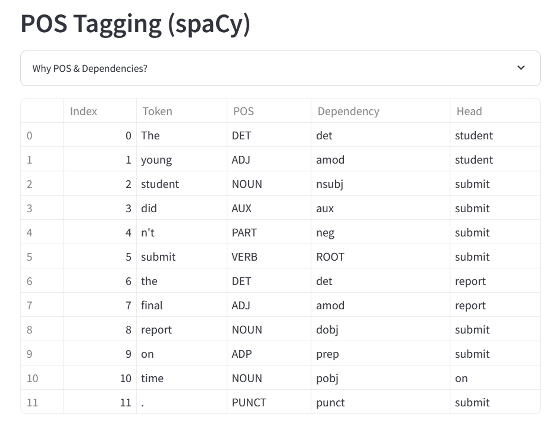
The meaning of the dependency strings is explained in the table at the bottom of the Meaning Machine page.
Each token is then converted into a list (vector) of hundreds of numbers that capture its meaning and context. The figure below shows each token in the example sentence visualized in two dimensions through
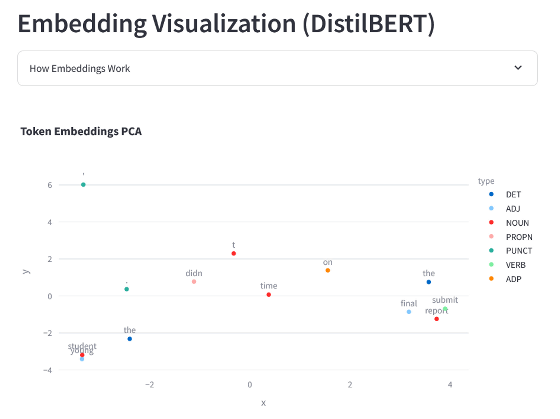
Below that is a tree showing the dependencies of each token, which shows which tokens depend on which other tokens, and what the whole picture means.
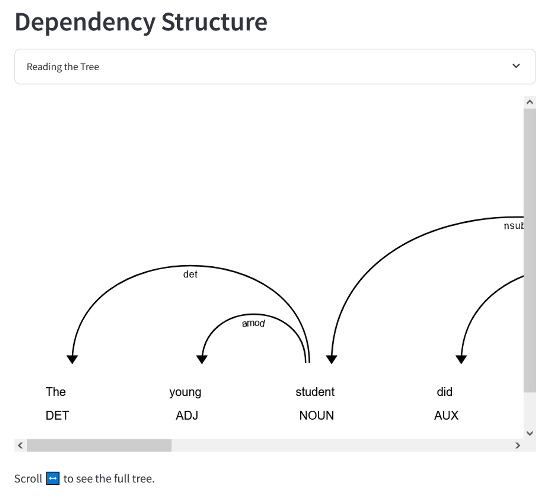
You can navigate through the dependencies by dragging the bar at the bottom of the diagram left and right.
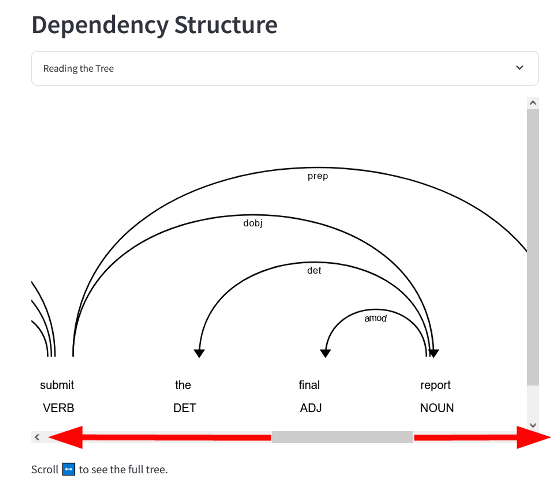
In Meaning Machine, you can enter any sentence you like into the input form at the top of the page to see how the large-scale language model converts each word into a token and how it captures the dependencies of the entire sentence.
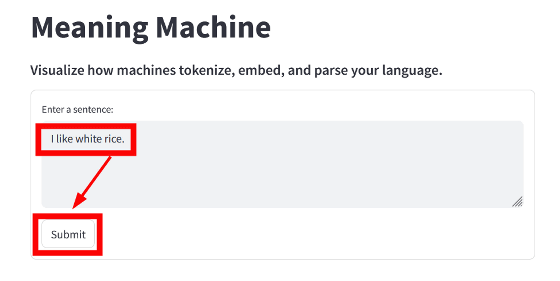
'These technical steps reveal something deeper: language models don't understand language the way humans do,' Hathcock said. 'They simulate language convincingly, but in a fundamentally different way. When you or I say 'dog,' we might recall the feel of fur, the sound of a bark, and even an emotional response. But when a large-scale language model sees the word 'dog,' it sees a vector of numbers formed by the frequency with which 'dog' appears near words like 'bark,' 'tail,' 'vet,' and so on. This is not wrong; it has statistical meaning. But this has no substance, no basis, no knowledge.'
In other words, large-scale language models and humans process language fundamentally differently, and no matter how human-like a response may be, there are no beliefs or goals. Despite this, large-scale language models are already widespread in society, creating people's resumes, filtering content, and sometimes even determining what is valuable. Since AI is already becoming a social infrastructure, Hathcock argued that it is important to know the difference in performance and understanding of large-scale language models.
Related Posts:
in Software, Web Service, Posted by log1h_ik