Using machine learning and nano 3D printing, a new material has been developed that combines the strength of steel with the lightness of polystyrene foam.
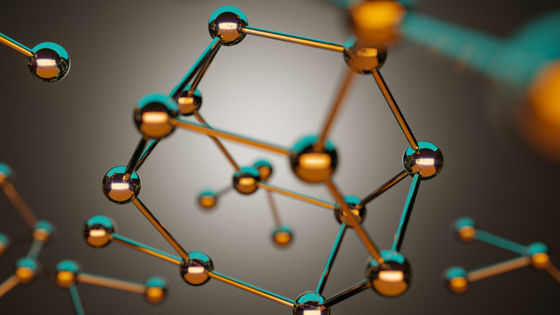
A research team led by the University of Toronto in Canada has announced that they have used machine learning to design a lightweight yet strong nanostructured material. It is expected that using this new structured material in aircraft and spacecraft bodies will significantly improve fuel efficiency while maintaining their strength.
Ultrahigh Specific Strength by Bayesian Optimization of Carbon Nanolattices - Serles - Advanced Materials - Wiley Online Library
Strong as steel, light as foam: Machine learning and nano-3D printing produce breakthrough high-performance, nano-architected materials - U of T Engineering News
https://news.engineering.utoronto.ca/strong-as-steel-light-as-foam-machine-learning-and-nano-3d-printing-produce-breakthrough-high-performance-nano-architected-materials/
'Nanostructured materials combine high-performance geometries at nanoscale sizes, such as triangular bridges, to exploit the 'smaller is stronger' effect and achieve the highest strength-to-weight and stiffness-to-weight ratios of any material,' says Peter Sells, a researcher in the University of Toronto's Department of Mechanical and Industrial Engineering.
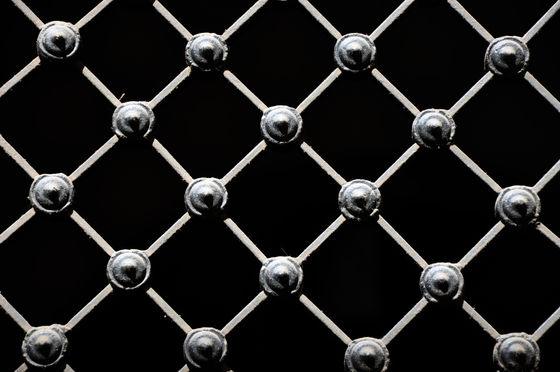
Sells explains that standard lattice shapes and geometric forms tend to have sharp intersections and corners that lead to
Sells and his team were working on this problem and realized that machine learning was the best way to solve it. So they teamed up with Professor Ryu Seung-hwa and doctoral student Yeo Jin-wook of the Korea Advanced Institute of Science and Technology (KAIST) to design an improved material.
To design the new nanostructures, the KAIST team employed a multi-objective Bayesian optimization (MBO) machine learning algorithm that learns from simulated shapes to predict optimal nanoscale shapes for enhanced stress distribution and improved strength-to-weight ratio.
Sells and his team then used a nanoscale 3D printer at the University of Toronto's Centre for Research and Applications of Fluid Technology (CRAFT) to print the results, creating a prototype of a lightweight carbon nanolattice material that combines ultra-high specific strength and scalability.
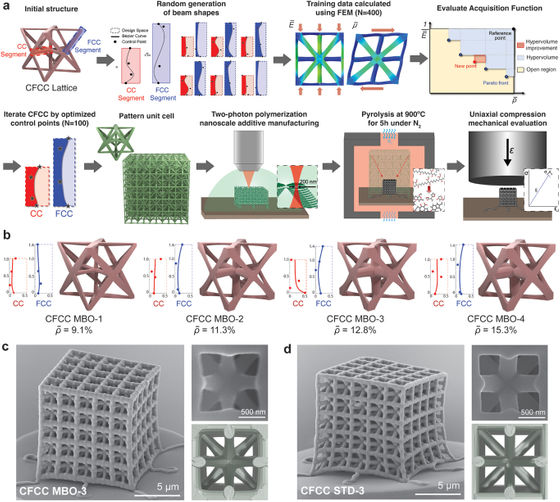
The nanostructured material that Sells and his team created is light enough to float on a soap bubble, yet is about five times stronger than titanium.
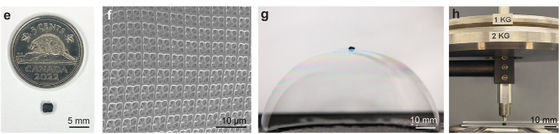
'This is the first time machine learning has been applied to optimize nanostructured materials, and we've been amazed at the improvements,' said Sells. 'The machine learning model not only found a shape that fit the training data, but also learned what changes to the shape would work and what changes would not work, and was then able to predict entirely new lattice shapes.'
The new material could be used to make ultra-lightweight parts for aircraft, helicopters and spacecraft, among other things. According to Sells, replacing titanium parts in aircraft with the new material could save 80 litres of fuel per year for every kilogram of material replaced.
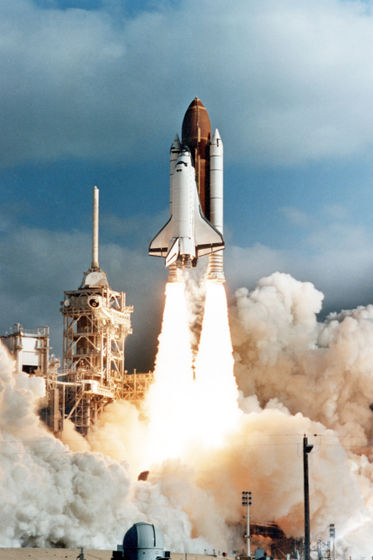
'Our next steps will focus on further improving the scale-up of these material designs and realizing cost-effective macro-scale components,' said Tobin Filleter, professor at the University of Toronto and leader of the research team. 'We will continue to explore new designs that allow us to further reduce the density of material structures while maintaining high strength and stiffness.'
Related Posts: